Introduction
Abdominal aortic aneurysm (AAA) is a common medical condition that affects approximately 5% of the general population and is one of the leading causes of death in developed countries [1]. AAAs can lead to rupture, a catastrophic event with a high mortality rate exceeding 80% [2]. Currently, the most prevalent and preferred treatment method for AAA is endovascular aortic repair (EVAR) [3]. However, this method is associated with specific complications, primarily due to its endovascular nature [4]. First described by White et al. [5], endoleak is a unique and potentially life-threatening complication in EVAR patients, characterised by persistent leakage of blood into the aneurysmal sac beyond the stent graft coating. Endoleak may cause further AAA sac expansion and subsequent rupture. Therefore, current guidelines advise lifelong diagnostic follow-up for EVAR patients [6].
The current guidelines include a list of diagnostic modalities used in post-EVAR surveillance, with ultrasound and computed tomography angiography (CTA) being the most significant. CTA remains the primary diagnostic tool for the follow-up of patients after EVAR [4]. It is easily accessible, reproducible, and allows for precise measurements and evaluations of potential complications with high diagnostic accuracy. Its variants, dual-energy CTA (DECTA) and photon-counting CTA (PCCTA), have also demonstrated high diagnostic value in post-EVAR surveillance [7-9]. DECT enables the creation of virtual monoenergetic images (VMIs), which replicate the attenuation values of an image captured at a single energy level (typically within the range of 40-200 keV). Low-keV images (40-70 keV) can better reveal subtle contrast enhancements due to greater beam attenuation from iodine [10]. Both imaging modalities have shown the ability to improve diagnostic accuracy in endoleak detection, providing a superior contrast-to-noise ratio (CNR) that facilitates the identification of subtle endoleaks [11]. The high diagnostic value of these images, exceeding that of classic reconstructions, has already been proven in research [12,13].
The recent boom of artificial intelligence (AI) in medicine has revolutionised the field, offering significant advancements in accuracy, efficiency, and personalised patient care. Recent advancements in AI algorithms in the medical field have made significant progress, especially within the field of radiology. Medical imaging, in particular, constitutes approximately 85% of FDA-approved AI programs as of 2023 [14]. AI has been proven to have high diagnostic capabilities for numerous tasks, among others, in oncology, paediatrics, dentistry, and vascular imaging [15-19]. AI has demonstrated superior diagnostic accuracy compared to clinical experts, streamlined workflows, and automated basic imaging analysis tasks [20]. The rapid evolution of AI in medical diagnostic imaging, particularly through deep learning technologies, has diversified its applications, making it a very promising tool in modern medical practice [5]. Despite very promising results, the use of AI in medical imaging is associated with multiple risks [21]. AI systems require rigorous validation to ensure their reliability across diverse clinical settings and patient populations. Inconsistent performance can undermine trust and effectiveness and, most importantly, can have a hazardous impact on patient health [22]. Therefore, continuous validation of AI algorithm function is mandatory for AI tool integration in clinical practice.
The detection of endoleaks following EVAR requires a time-consuming review of multislice CTA images by human readers. The process can be time-consuming and prone to potentially life-threatening errors. Additionally, with the increasing number of post-EVAR patients and the necessity for regular imaging, the number of examinations is constantly increasing. Therefore, the application of AI tools could streamline the diagnostic process and increase the accuracy of assessments. Despite the exponentially growing number of studies on AI utilisation in medical imaging, the automated evaluation of EVAR outcomes by AI remains a topic with few published studies to date. Thus, the application of AI in detecting leaks, as well as the impact of VMI reconstruction on AI diagnostic parameters, seems to be very interesting.
Recent advances in the application of artificial intelligence to medical imaging have been significant and have led to many new applications, including the detection of endoleaks. One of the recent applications is the PRAEVAorta®2 software (Nurea, Belges,France), which enables the reconstruction and visualisation of arteries and veins based on DICOM images. Based on tests conducted by the company, this software significantly accelerates endoleak detection through segmentation and achieves performance statistics comparable to those of human readers [23].
The aim of this study was to assess the diagnostic accuracy of the aforementioned AI tool for evaluating endoleaks in post-EVAR CTA with linearly blended and VMI reconstructions.
Material and methods
The Ethics Committee of Collegium Medicum at Nicolaus Copernicus University in Torun, Poland, approved the study (no. 440/2018). The study was carried out in compliance with the Declaration of Helsinki and relevant guidelines. All participants provided written informed consent.
Population
The study involved 95 consecutive patients who underwent EVAR procedures and were referred for 95 CTAs performed between August 2019 and December 2020. A follow-up examination was conducted for every patient one month after the stentgraft implantation procedure. The inclusion criteria consisted of presence of an AAA and an age over 18 years. The exclusion criteria were known severe adverse reactions to iodinated contrast media, impaired renal function (glomerular filtration rate < 30 ml/min), and severe motion artifacts.
CT scanning protocol
All CT scans were obtained using a dual-energy fast-kVp switching scanner (Discovery 750 HD, GE Healthcare, Milwaukee, WI, USA). The standard examination protocol consisted of 3 phases: one nonenhanced phase and 2 postcontrast dual-energy acquisitions (arterial and 60-s delayed phases). Both postcontrast phases were acquired using the following tube parameters: tube voltage, 80-140 kV; tube current, 360 mAs; pitch, 0.985:1; slice thickness, 0.625 mm; and 35 cm DFOV. Intravenous administration of 80 mL of iohexol (350 mg I/ml), a nonionic iodine contrast agent, through the peripheral vein at the forearm, was performed at a rate of 4 ml/min. The contrast agent was followed by a saline bolus chaser. A bolus tracking tool was used to trigger the start of arterial acquisition once the region of interest (ROI) in the proximal descending aorta exceeded 125 HU.
Image reconstruction
The data acquired during the dual-energy scan in the arterial phase were reconstructed as follows:
Linearly blended images (a fusion of 70% 140 kVp and 30% 80 kVp datasets) closely resemble the traditional CT scan obtained with a single energy of 120 kVp.
40 keV VMI.
All measurements were performed using a dedicated GE Healthcare console (GSI Viewer, Advantage Workstation Release 4.7, GE Healthcare).
Evaluation of human readers
Both datasets were independently assessed by 2 readers with at least 5 years of experience in CTA assessment. The endoleak types were evaluated according to the classification proposed by Karkkainen et al. [24]. Both readers were blinded to each other’s and the AI’s results.
The readers assessed the images using a biphasic protocol consisting of a true noncontrast and one postcontrast arterial phase. Since the AI program did not utilise the 60-second delayed phase in its assessment, it was not used in this study. After the reading sessions, the images were jointly evaluated, and a consensus on the presence and type of endoleak was reached. The consensus sequence subsequently served as the reference standard.
AI evaluation
The AI assessment of the collected datasets was carried out using PRAEVAorta®2 software (Nurea, Bègles, France). According to the program’s protocol, only images acquired in the arterial phase of the examination were uploaded to the cloud-based AI platform. Two sets of images were uploaded:
The program automatically generated reports on the presence of the endoleaks.
Results
Patient population
One patient from the initial study group was excluded because they failed to meet our inclusion criteria (EVAR procedure due to aortic dissection). Ultimately, a total of 94 patients (14 women, 80 men; mean age 71.5 years, range 55-89) were included in the study. All CT scans were performed 30 days after stent graft implantation. In 53 patients the scan area was limited to the abdominal cavity and pelvis, and in 42 patients the scan area also included the thorax. Sixty-eight patients underwent classic endovascular stent graft implantation for an AAA, and 26 patients underwent branched or fenestrated EVAR.
Evaluation of human readers
The first study session included a protocol consisting of TNC and arterial phase LB images and revealed the presence of 44 endoleaks in 31 patients (32.9% of the total number of patients). Among the identified endoleaks, the most frequently diagnosed were type II endoleaks, which were diagnosed 18 times. Nine patients had at least 2 endoleaks: 4 patients with type III endoleaks and 2 with type II endoleaks; 2 patients with 2 type II endoleaks; 2 patients with type Ia and type III endoleaks; and one patient with 3 type II endoleaks.
Session II of the study included an evaluation of the TNC and an arterial phase 40 keV VMI. Both readers identified 50 endoleaks. Ten patients who had an endoleak in session II were not diagnosed with endoleak in session I with LB images. Of the 10 additional endoleaks detected in the VMI protocol, 8 type II endoleaks and 2 type III endoleaks were identified.
AI evaluation
Because the AI tool assigns data to 2 classes, the problem of endoleak detection can be treated as a binary classification. Four measures were defined as follows:
TP – true positives: patients with endoleak classified with endoleak by AI tool;
FP – false positives: patients without endoleak classified with endoleak by AI tool;
FN – false negatives: patients with endoleak classified as no endoleak by AI tool;
TN – true negatives: patients without endoleak classified as no endoleak by AI tool.
AI tool performance against human readers was evaluated using accuracy, precision, recall, and F1-score with the formulas specified in paper by Saito and Rehmsmeier [25].
The first session of the study evaluated the images acquired in the arterial phase – LB images. In contrast to human readers, an AI program did not show the number of endoleaks diagnosed in each patient. Patients were diagnosed regarding the presence of endoleaks. If they were present, the program reported the range of axial slices with endoleak presence. A greater number of endoleaks were not reported. In total, 34 patients were diagnosed with endoleaks. The types of endoleaks were not reported. AI evaluation of the LB images showed a total of 34 patients with endoleaks. The confusion matrix provides a detailed breakdown of the AI program’s predictions compared to the reference standard. The confusion matrix is depicted in Figure 1.
The second aim of the study was to assess whether 40 keV VMIs influence the diagnostic accuracy of the program. The second session utilised 40 keV VMI images acquired in the arterial phase of the examination. However, after uploading the 40 keV datasets, the program was unable to correctly define the lumen of the stentgrafts. Therefore, we were unable to conduct further analyses.
Evaluation metrics
Table 1 presents the key metrics calculated from the confusion matrix, including accuracy, precision, recall, F1 score, and the area under the ROC curve (AUC).
Table 1
Summary of AI program diagnostic accuracy metrics in endoleak detection (LB images)
Accuracy | Precision | Recall | F1 Score | AUC |
---|---|---|---|---|
78.72% | 67.65% | 71.88% | 69.70% | 0.77 |
The ROC curve, shown in Figure 2, illustrates the trade-off between the true positive rate and false positive rate for the AI program, with an AUC of 0.77, indicating the overall performance of the model.
Discussion
The results of this study demonstrate the moderate diagnostic accuracy of the evaluated AI program in endoleak detection in post-EVAR patients. The AI program evaluated in this study showed a high level of accuracy (78.7%), balanced precision (67.6%), and recall (71.9%), with an F1 score of 69.7% and an AUC of 0.77. These findings show that the program can aid in endoleak detection; however, a significant proportion of misdiagnoses cause us to conclude that it is not yet ready for commercial use.
Recent advancements in AI have introduced machine learning (ML) and deep learning (DL) tools to potentially enhance the accuracy and efficiency of endoleak detection. AI models, particularly deep neural networks, have shown high accuracy, precision, and recall in detecting endoleaks, often outperforming general radiologists and matching the performance of subspecialists [26-28]. The study by Talebi et al. [26] evaluated the diagnostic performance of the Endoleak Augmentor, a custom-designed ML model for endoleak detection. The authors demonstrated the model’s high performance, with an accuracy, precision, and recall of 90%, 83%, and 100%, respectively. However, the study evaluated only 20 CTAs, 10 of which contained endoleaks. Hahn et al. [27] evaluated the performance of the ResNet-50 convolutional neural network (CNN) in endoleak detection on individual axial slices. The CNN automatically assessed AAA and endoleak volumes. The model showed an area under the receiver operating characteristic curve of 0.94 ± 0.03, with an optimised accuracy of 0.89 for endoleak detection. Additionally, the program precisely measured the AAA and endograft volume (Dice coefficient, 0.95 ± 0.2); however, the endoleak volume measurements were much less accurate (Dice coefficient, 0.53 ± 0.21). The authors concluded that the proposed CNN model can accurately detect and measure endoleaks after EVAR, potentially improving surveillance after the procedure. Kordzadeh et al. [28] evaluated the applicability of AI tools for the prediction, pattern recognition, and modelling of post-EVAR complications [28]. The accuracy of the training, validation, and predictive ability of the ANN in detecting endoleaks varied between types of endoleaks and ranged from 82% to 96%, with a predominance of values above 90%. Despite these promising results, our study showed lower diagnostic accuracy of the tested AI program, indicating the need for refinement. Figure 3 shows obvious endoleaks not diagnosed by the AI program. It should be noted, however, that the aforementioned AI tools were research projects trained on sets of images acquired in specific centres. The products tested in this research showed less diagnostic accuracy; however, the datasets used were probably different from the training data. This factor might have contributed to the lower diagnostic accuracy metrics. Our study evaluated the precommercial use of the program. We believe that some algorithm refinements might improve the program’s diagnostic accuracy.
Figure 3
Endoleaks unidentified by the AI program: A – endoleak type Ia, B – endoleak type Ib, C – endoleak type II, D - endoleak type III
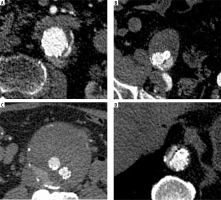
The tested AI tool was an experimental component of the PRAEVAorta®2 software, a fully automated tool designed for the segmentation and analysis of infrarenal AAAs using CT images. This tool aims to improve the accuracy and efficiency of diagnosing and planning treatments for AAAs by providing detailed anatomical characteristics. To date, a few studies have been published showing its high reliability in these tasks [23,29,30]. The authors demonstrated the program’s high accuracy for aneurysm detection, delineation, and volumetric analysis. In contrast to our results, Coatsaliou et al. [30] reported the outstanding diagnostic accuracy of PRAEVAorta®2 for detecting endoleaks in a group of 100 patients. The program achieved a sensitivity of 89.47%, specificity of 91.25%, PPV of 90.67%, and NPV of 90.12% in detecting endoleaks. Such large differences in diagnostic accuracy probably stem from differences in the input datasets. This possibility is indicated by the fact that the program was unable to correctly segment structures on 40 keV datasets. Therefore, we assume that LB images might not be optimal input data for the algorithm. This emphasises the necessity, as already indicated in the literature, of training the algorithm on many different datasets [21].
The follow-up of patients post-EVAR is crucial for monitoring complications such as endoleaks, aneurysm growth, and other morphological changes [6]. Studies have shown that AI holds promise in enhancing the accuracy and efficiency of these follow-up processes, not directly including automatic endoleak detection. AI-driven models can predict postoperative outcomes, including mortality and complications after EVAR, by analysing large datasets to identify predictive patterns [31,32]. AI tools such as augmented radiology for vascular aneurysm (ARVA) provide accurate preoperative and postoperative assessments of aortic diameter, reducing the need for time-intensive manual measurements [33]. Moreover, AI tools might improve endoleak visualisation. A study by Kazimierczak et al. [34] evaluated the image quality parameters and diagnostic value of the DL-model denoising tool ClariCT.AI (ClariPI, Seoul, South Korea) for detecting endoleaks. The authors showed substantial improvements in objective and subjective image quality properties in DL denoised images. Taking into account all the presented data, we believe that the increasing use of AI tools in post-EVAR surveillance is inevitable.
The classic CTA examination protocol includes 3 phases: one unenhanced phase and 2 postcontrast (arterial and 60-second delayed) phases. The unenhanced phase distinguishes endoleaks from hyperdense areas such as calcifications, embolic materials, and coils [35]. Common diagnostic protocols use 2 postcontrast phases, with the delayed phase crucial for detecting low-flow endoleaks not visible in the early phase [36,37]. Furthermore, some researchers suggest extending the delayed phase to 300 seconds to identify additional endoleaks that remain undetected in the standard delayed phase [38]. Therefore, in our opinion, the approach based on diagnosing leaks solely from the arterial phase of the examination is controversial and may result in a large number of false positive results (due to the misdiagnosis of calcifications as the endoleak) and false negative results (due to the presence of low-flow endoleaks).
Studies indicate that VMIs improve the diagnostic accuracy of endoleak detection [39,40]. The differences in the number of endoleaks diagnosed in both LB and 40 keV CMIs stem from improved contrast and endoleak attenuation of VMI images. These findings were already shown in our 2023 study [12]. Similar results were shown by other researchers who evaluated the utilisation of low-keV VMIs in endoleak detection [39,40]. The scientific literature indicates other potential benefits associated with the use of DECT in EVAR patient surveillance, such as improved stent visualisation and the possibility of reducing the radiation dose by replacing the TNC phase with virtual noncontrast reconstructions [12,37,41-47]. Therefore, we regret that we were unable to assess the diagnostic accuracy of the AI program on VMI images, and we believe that the joint use of AI and DECT reconstructions might provide better diagnostic accuracy and thus ensure better patient outcomes.
This study has several limitations that need to be addressed. First, the sample size of 94 patients, while sufficient for preliminary analysis, is relatively small and may not fully represent the broader population of post-EVAR patients. Second, the AI tool evaluated in this study showed limited effectiveness with 40 keV VMIs, suggesting that the type of input data significantly affects its performance. The inability to process these images correctly indicates that the AI algorithm needs further refinement to handle various image types effectively. Finally, the inability of AI programs to classify the types of endoleaks significantly limits their clinical utility. Accurate classification of endoleak types is essential for appropriate patient management, and the lack of this feature reduces the effectiveness in clinical practice.
Conclusions
In conclusion, this study highlights the potential and current limitations of AI in detecting endoleaks using dual-energy CTA. The evaluated AI program demonstrated moderate diagnostic accuracy, with an accuracy rate of 78.7%, a precision of 67.6%, and a recall of 71.9%. Despite these promising findings, the AI tool failed to achieve the high diagnostic performance necessary for reliable clinical application due to a significant number of false positives and negatives.